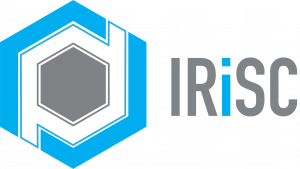
Interdisciplinary Research Group in Socio-technical Cybersecurity
An Analysis of Cholesteric Spherical Reflector Identifiers for Object Authenticity Verification
Abstract:
Arrays of Cholesteric Spherical Reflectors (CSRs), microscopic cholesteric liquid crystals in a spherical shape, have been argued to become a game-changing technology in anti-counterfeiting. Used to build identifiable tags or coating, called CSR IDs, they can supply objects with unclonable fingerprint-like characteristics, making it possible to authenticate objects. In a previous study, we have shown how to extract minutiæ from CSR IDs. In this journal version, we build on that previous research, consolidate the methodology, and test it over CSR IDs obtained by different production processes. We measure the robustness and reliability of our procedure on large and variegate sets of CSR IDs’ images taken with a professional microscope (Laboratory Data set) and with a microscope that could be used in a realistic scenario (Realistic Data set). We measure intra-distance and interdistance, proving that we can distinguish images coming from the same CSR ID from images of different CSR IDs. However, without surprise, images in Laboratory Data set have an intra-distance that on average is less, and with less variance, than the intra-distance between responses from Realistic Data set. With this evidence, we discuss a few requirements for an anti-counterfeiting technology based on CSRs
Authors:
Mónica P. Arenas , Hüseyin Demirci and Gabriele Lenzini
Publication date:
Received: 23 January 2022 / Revised: 17 February 2022 / Accepted: 21 February 2022 / Published: 24 February 2022
Published in:
Machine Learning & Knowledge Extraction, selected as the cover page of Volume 4, Issue 1.
Reference:
Arenas, Mónica P., Hüseyin Demirci, and Gabriele Lenzini. 2022. "An Analysis of Cholesteric Spherical Reflector Identifiers for Object Authenticity Verification" Machine Learning and Knowledge Extraction 4, no. 1: 222-239.
Get in touch with us
SnT – Interdisciplinary Centre for Security, Reliability and Trust
Maison du Nombre, 6, avenue de la Fonte L-4364 Esch-sur-Alzette
info-irisc-lab@uni.lu